Is AI Ready for DevOps Alert Management?
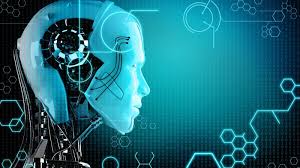
Limited Time Offer!
For Less Than the Cost of a Starbucks Coffee, Access All DevOpsSchool Videos on YouTube Unlimitedly.
Master DevOps, SRE, DevSecOps Skills!
Source – it.toolbox.com
DevOps requires monitoring of processes to ensure the adequate performance of systems and software. No one is going to sit in front of an updating dashboard continuously looking at system performance metrics. Likewise, and more commonly, messages or emails sent from systems or people about problems can be ignored easily.
DevOps teams need systematic means of monitoring and alerts to problems as they occur. The threshold needs to be such that they see important issues immediately, without inboxes getting cluttered with too many messages. This sounds like a great use case for artificial intelligence (AI).
AI can be used to trigger alert messages on critical system conditions or problematic errors while excluding or summarizing lower-level issues. To believe the AI hype, you would think some sentient agent would be roaming an enterpriseās network and applications, sniffing out problems before they occur.
Reality Check
AI doesnāt really operate that way. Much of the hype around AI makes it sound like a generalized super-sentient system is coming.
In reality, AI uses historical data to discern patterns and trends. The direct implication is that you must have historical data. Many DevOps situations provide new functionality or new systems. Often, no historical database is available to train the AI models.
Similar projects from the past may have data, but most projects have specific incidents and performance metrics, unlike previous DevOps projects.
Making a Fit
AI is such a trendy topic that many IT organizations and teams feel they have to do something with it. Despite the lack of historical data, AI could have an immediate place in alerts for DevOps.
As more and more enterprise computing functions move to cloud services, the volume of automated alerts is exploding. Hosted services try to err on the side of caution with system alertsābetter to notify too much than miss a critical problem. Their default settings send too much information to the subscribing IT department.
Here is the opportunity to apply AI to the alert management process.
Aggregating and Learning
AI needs historical data. You likely already have a set of alerts coming from existing systems that, with a little massaging, could be made into a database for AI algorithms to learn from.
Hand coding the action level or some other classification of previous messages lets AI systems try to pick out patterns and trends associated with different severity levels. Aggregating these from various projects is the basis for a generalized smart alert system.
Imagine all alerts from many different services and vendors go to a common inbox (inbox in this context is just a centralized area). Using the hand-coded action levels for previous alerts, an AI system can be trained to look for specific words, phrases, codes, times, and so on.
Associations between all factors might be used to create a secondary alerting system. This secondary alert management system triages all of the incoming messages from all the different services. The AI system might relay critical messages immediately, wait on less severe problems to occur repeatedly, or group planned maintenance messages into daily summaries, for example.
Communications Management
The AI system can also use multiple channels to communicate with key personnel. For the same message, some might receive immediate alerts via text message on a mobile device while other individuals get the same notification on Slack or Microsoft Teams, and others might see the message in their email inbox.
Although this kind of work could be hardcoded based on agreed-upon rules, the AI system does have an advantage in being able to rerun models to learn from new information. Coding new rules into a database or business intelligence system takes time and effort. Using a self-updating AI system requires less work in the short term.
An AI-centered alert management system is not going to be perfect, but it might let DevOps teams worry less about notifications.